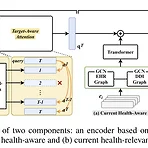
본 논문 리뷰는 개인적인 학습 내용을 바탕으로 작성된 것으로, 일부 내용에 오류가 있을 수 있습니다. 논문 정보 Kim, T., Heo, J., Kim, H., Shin, K., & Kim, S. W. (2024, March). VITA:‘Carefully Chosen and Weighted Less’ Is Better in Medication Recommendation. In Proceedings of the AAAI Conference on Artificial Intelligence (Vol. 38, No. 8, pp. 8600-8607). VITA: 'Carefully Chosen and Weighted Less' Is Better in Medication RecommendationWe addre..
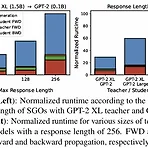
본 논문 리뷰는 개인적인 학습 내용을 바탕으로 작성된 것으로, 일부 내용에 오류가 있을 수 있습니다. 논문 정보 Ko, J., Kim, S., Chen, T., & Yun, S. Y. (2024). Distillm: Towards streamlined distillation for large language models. arXiv preprint arXiv:2402.03898. DistiLLM: Towards Streamlined Distillation for Large Language ModelsKnowledge distillation (KD) is widely used for compressing a teacher model to a smaller student model, reducing it..
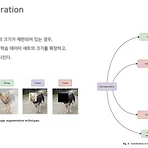
논문 정보Imrus Salehin, Md. Shamiul Islam, Pritom Saha, S.M. Noman, Azra Tuni, Md. Mehedi Hasan, Md. Abu Baten, AutoML: A systematic review on automated machine learning with neural architecture search, Journal of Information and Intelligence, Volume 2, Issue 1, 2024, Pages 52-81, ISSN 2949-7159, https://doi.org/10.1016/j.jiixd.2023.10.002.
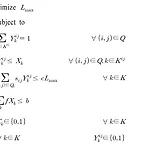
논문 정보 로드밸런싱을 위한 전기차 충전소 입지선정 문제In South Korea, Jeju Island has a role as a test bed for electric vehicles (EVs). All conventional cars on the island are supposed to be replaced with EVs by 2030. Accordingly, how to effectively set up EV charging stations (EVCSs) that can charge EVs is awww.kci.go.kr1. Introduction본 논문은 부하 평준화 기법(load balancing method)을 활용한 최적 입지선정 모델을 제시하였다.해당 모형은 전체 수요를 충족시킬 수 ..
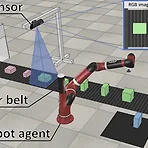
본 연구는 3D BPP를 해결하기 위해 Actor-critic 구조를 가진 DRL을 활용하여 3D BPP 문제를 해결하는 연구이다.논문 정보 Online 3D Bin Packing with Constrained Deep Reinforcement LearningWe solve a challenging yet practically useful variant of 3D Bin Packing Problem (3D-BPP). In our problem, the agent has limited information about the items to be packed into the bin, and an item must be packed immediately after its arrival without buffe..
AdaBoost를 만들기 위한 구조화를 실습과 패키지를 활용하여 AdaBoost를 실습해본다. AdaBoost 메서드fit(X, y): 설명 주어진 입력 데이터 X와 타겟 레이블 y를 사용하여 AdaBoost 모델을 학습 입력X: 학습 데이터(특성 행렬). 크기: (n_samples, n_features). y: 타겟 레이블. 크기: (n_samples,). 역할지정된 약한 학습자(예: 결정 스텀프)를 반복적으로 학습시키고, 각 학습자의 가중치를 계산하여 최종 모델을 구성 predict(X): 설명 학습된 AdaBoost 모델을 사용하여 새로운 데이터 X에 대한 예측을 수행 입력X: 예측할 데이터(특성 행렬). 크기: (n_samples, n_features). 출력각 샘플에 대한 예측된 클래스 ..
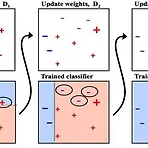
에이다 부스트(AdaBoost) 알고리즘에 대해 알아본다. 에이다 부스트오류 데이터에 가중치를 부여하면서 부스팅을 수행하는 알고리즘으로, AdaBoost 학습 단계에서 모델이 잘못 예측한 샘플에 더 큰 가중치를 부여하여 더 잘 학습하도록 유도한다. 초기에는 모두 동일한 가중치를 가지며, 단계가 진행될수록 잘못 예측한 샘플의 가중치를 점진적으로 증가시켜 이전 학습기들이 잘못 예측한 부분을 보완하도록 유도한다. Input : Dataset $ D = \left\{ (x^1, y^1), \cdots ,(x^N, y^N) \right\},$ $y^{(i)}\in \left\{-1, 1 \right\}$ $\textrm{for}$ $i = 1, \cdots , N$ Step0 : 모든 $i = 1, \cdo..
- Total
- Today
- Yesterday